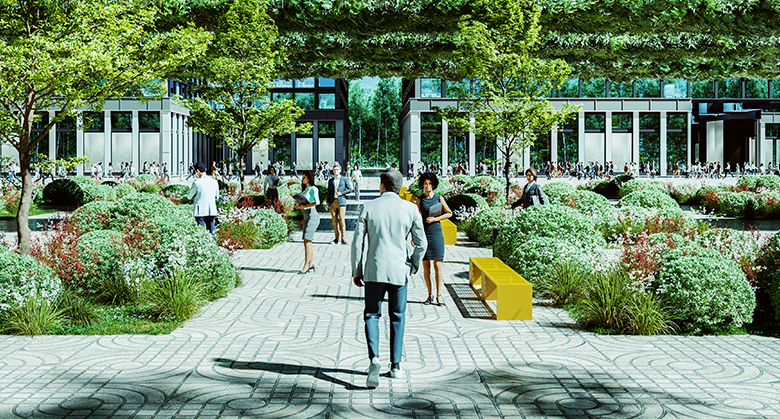
A Fresh Approach to Evaluate Walkability Using Machine Learning
In the United States, city officials and developers have long relied on conventional traffic engineering and roadway design standards that prioritize vehicle movements, resulting in car-centric cities. However, the transportation paradigm is rapidly changing with a new focus on walkability and other active transportation modes. This shift requires new standards that prioritize walkability and promote livable communities.
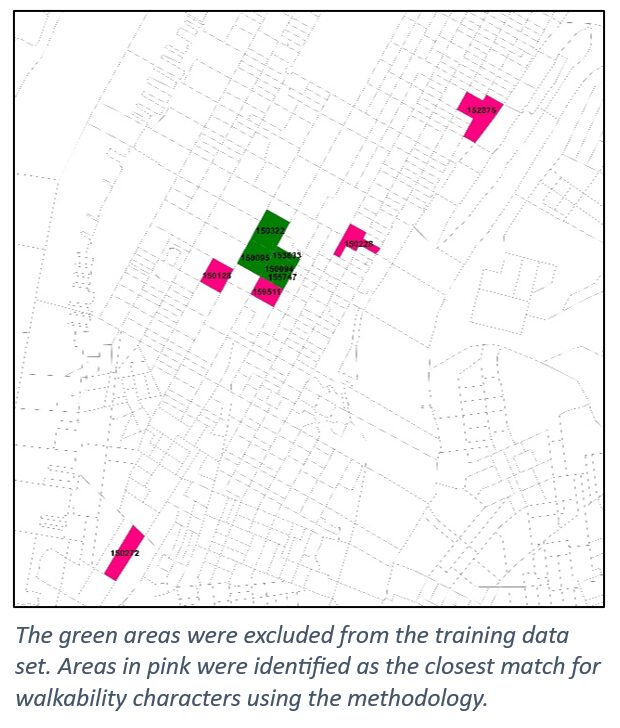
To address this need, researchers developed an innovative methodology using a k-nearest neighborhood (KNN) machine learning algorithm that allows decision-makers to visualize how their planned development will function in terms of walkability. By utilizing existing developments among 220,740 U.S. Census Block Groups (CBG), the algorithm enables planners to identify the closest match to their development plan. By assessing the walkability of the closest match, planners can make informed decisions about their development before it is implemented.
The methodology was validated by testing four CBGs in New York City, which were intentionally excluded from the training data. The results identified the closest match to be four CBGs within the same vicinity. Following this, three different developments in Dubai were evaluated by identifying their closest matches within the CBGs, demonstrating the methodology’s potential for use in diverse urban contexts. The indicators used in the algorithm include intersection density, land use mix, proximity to public transit, and land use density, which are essential to ensure that the development is pedestrian-friendly and encourages active transportation.
Additionally, planners can adjust the walkability indicators of a proposed master plan development until their development matches and functions like their desired Census Block Group. This careful process of “what if” analysis using the tool ensures that the final plan meets the client’s vision.
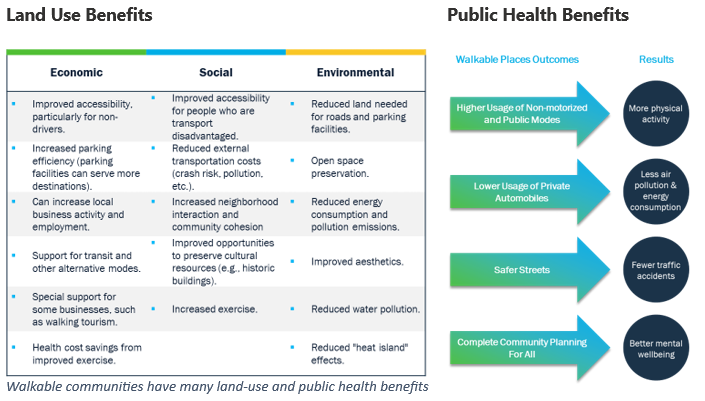
As urbanization continues to rise, it is crucial for decision-makers to prioritize sustainable and walkable communities. The use of innovative methodologies, such as this machine learning algorithm, is a step in the right direction toward creating livable neighborhoods that prioritize active transportation. By taking a holistic approach to urban planning and engaging with local communities, we can create thriving cities that promote wellness and sustainability for all residents.